Workshop on Responsibly Building the Next Generation of Multimodal Foundational Models
NeurIPS 2024
Date: 14th December, Location: Meeting Room 217 - 219, Vancouver, Canada
Introduction
In recent years, the importance of interdisciplinary approaches focusing on multimodality (language+image+video+audio) has grown exponentially, driven by their impact in fields such as robotics. However, the rapid evolution of these technologies also presents critical challenges regarding their design, deployment, and societal impact. Large Language Models (LLMs) sometimes produce "hallucinations," and Text-to-Image (T2I) diffusion models can inadvertently generate “harmful content.” These models pose unique challenges in fairness and security. Addressing these challenges preemptively is crucial to breaking the cycle of reactive measures and reducing the substantial resource burden associated with post-hoc solutions. These preemptive measures can be applied at various stages, such as dataset curation and pre-training strategies, while maintaining resource efficiency to promote more sustainable development of generative models.
Our workshop aims to provide a platform for the community to openly discuss and establish responsible design principles that will guide the development of the next generation of generative models. The goals of this workshop are to:
- Discuss methodologies that enhance the reliability of multimodal models, tackling key issues such as fairness, security, misinformation, and hallucinations.
- Enhance the robustness of these models against adversarial and backdoor attacks, thereby securing their integrity in adversarial environments.
- Identify the sources of reliability concerns, whether they stem from data quality, model architecture, or pre-training strategies.
- Explore novel design principles emphasizing responsibility and sustainability in multimodal generative models, aiming to reduce their extensive data and computational demands.
Keynotes
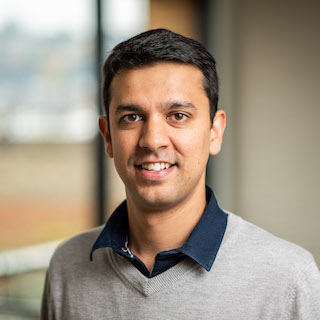
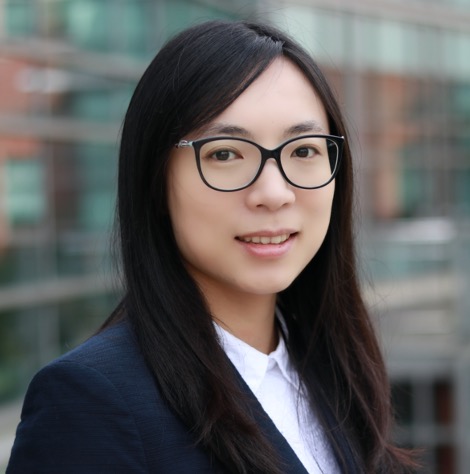
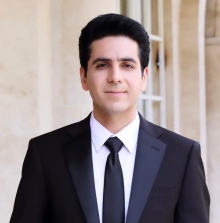